Artificial Intelligence is changing software testing. It helps to make more quality software while using fewer resources (or spending them more wisely).
Yet, what will we see if we look into it a bit closely? Under 40% of skilled professionals in QE and QA rely on AI tools for their important tasks. General dependence is the following: the more experienced the professional, the less they are inclined to use AI.
Why?
That’s the question — but probably for a dedicated article. Now, just recall how doubtful people were about smartphones. Touchscreen instead of buttons? No way! Fast forward to today — could you imagine your online chit-chatting hitting those buttons?
Another example is how AI integrates into our routines through personalized digital assistants like Siri and Alexa, which have become common tools for managing daily tasks.
From voice commands and personalized recommendations to smart home control — as we got used to it, AI in software testing has time to change our minds as well. As of now, it automates repetitive tasks, learns from data patterns, and gives actionable, well-served insights into your potential strategy refinement.
Let’s debunk some AI-powered testing myths and demonstrate the value of Artificial Intelligence in the modern quality assurance process.
Myth 1: AI will replace human testers
You can’t escape the concern that AI will render humans of almost any specialization, particularly developers and software testers. There are many AI tools for broad usage and specifically for coding. The recent release of the o3-mini high model from OpenAI shows that it beats many scientists, not just developers, in deep problem-solving.
The reality
As of 2025, we are not talking about complete substitution for human testers, but rather about complementing them by automating repetitive and time-consuming tasks.
It just allows us to focus on more complex and strategic activities. We all know: Machines don’t have intuition and empathy (yet), as well as creativity. Autonomous QA tools can efficiently handle regression testing, but humans still excel in exploratory testing, usability assessments, and understanding nuanced user experiences.
So, just imagine how far you can lead your software if you enable testers to use modern technologies, including Artificial Intelligence.
Example
Your QA team runs a lot of constant tests. For example, regression suites. OwlityAI automates and streamlines this process and saves months compared to manual testing.
This way, testers can pay more attention to exploratory and usability testing, more creativity- and intuition-required areas. Previously manual regression test suite took several days to execute; Now it is completed in hours with OwlityAI.
Key takeaway: AI doesn’t replace human testers; it helps them work efficiently and focus on higher-value tasks, simultaneously boosting their professional value and growth.
Myth 2: AI is too complex for teams to implement
Integrating AI-powered tools into existing workflows is so daunting…right? Not quite true. Modern AI testing tools don’t require extensive technical expertise and are approachable even for non-tech staff.
The reality
These next-gen instruments are designed with growth in mind. That’s why vendors have built all possible scenarios in their products. That is, vendors took into account that startups may not have specialists with domain expertise in software testing and that enterprises, for example, will not have much time to maintain and optimize the current test library.
Modern AI tools usually take minimal effort with little to no coding experience. No disruptions, no need for specialized skills.
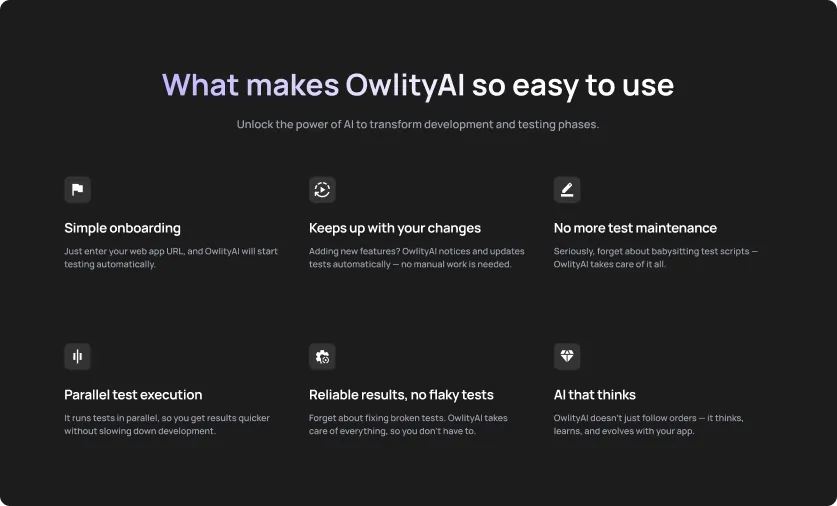
Key takeaway: AI tools are accessible and straightforward to implement — suitable for teams regardless of their prior AI experience.
Myth 3: AI testing tools can’t handle complex applications
Sophisticated stock exchange app, strictly regulated fintech solution, or medtech software with many integrations — AI can’t help but fail in these niches. A common misconception, indeed. Skepticists think that this technology is only suited for simple, repetitive test cases and struggle with complex apps.
The reality
It’s more like vice versa. Traditional scripted automation will struggle with API, dynamic interfaces, and intricate dependencies within a particular app. On the other hand, autonomous testing tools adapt to dynamic environments and don’t need constant oversight due to their self-healing capabilities and advanced analyzing features.
How and where AI excels:
- Scanning → analysis → test case generation: The principle behind an AI testing tool is simple: it keeps scanning the app, analyzes its UI and backend structure, and automatically generates relevant test cases.
- Self-healing: The tool detects UI changes – element shifts, renaming, or repositioning – and updates test scripts. Your test library will always be up-to-date and robust.
- Edge case identification: Next-gen tools operate incomparably larger data sets; that’s why they usually better uncover edge cases and unpredictable behavior.
- API and microservices testing: AI-powered testing frameworks validate interactions between microservices, ensuring smooth communication and performance across distributed systems.
- Handling real-world conditions: Autonomous QA tools simulate various network conditions, device configurations, and user interactions. It’s another misconception that AI can’t create real-world situations. It can.
Key takeaway: AI perfectly handles complex testing environments — a great asset for testing sophisticated applications with dynamic elements, integrations, and evolving user interactions.
Myth 4: AI is expensive and only for large enterprises
With all that buzz in the media about OpenAI’s, Microsoft’s, and Musk’s investments in AI and the latest US agreement on a USD 500B-worth program, many people are sure AI costs an arm and a leg. All prejudices stem from this and make AI impractical for startups and SMBs.
The reality
AI testing is no longer a luxury reserved for large enterprises. Autonomous testing instruments effortlessly scale, adjusting to the size of our business. Usually, they have flexible pricing and allow organizations to pay based on their testing needs — no need for extra commitment.
Cost-effectiveness of AI:
- Reduced manual effort: The traditional approach involves significant time for script creation and maintenance. On the other hand, AI automates their generation, execution, and updates. Modern testing tools cut costs by 85+% on average.
- Faster test cycles: Without manual checkpoints and redundant moves, testing time drops from days to hours. No delays, faster releases, faster time to market.
- Wider test coverage: Artificial Intelligence operates a larger amount of data, so it considers more possible user paths and usage cases. This translates into a higher test coverage with fewer resources.
- Wise resource allocation: Small QA teams can achieve enterprise-level testing capabilities without scaling headcount.
Example
A mid-sized SaaS company opted for OwlityAI to replace its manually maintained regression suite. They chose the core plan to test the waters. With this advanced automation, they reduced their QA team’s regression testing workload, cutting testing costs by 93%.
Key takeaway: AI testing is a smart, cost-effective investment that scales with business needs. It acts as a robust business partner on your testing side — moves with cost reduction and efficiency gains in mind.
Myth 5: AI is not reliable or mature enough
AI in QA is rather at an experimental level than a mature solution. It screws a lot and is not yet suitable for critical quality assurance tasks. A common thought, is that true?
The reality
Contrary to this belief, AI in software testing has matured significantly. Many tech pros call AI the best thing since sliced bread. Its results are quite accurate and reliable.
84% of surveyed software executives stated that they began AI adoption six months to five years ago. And 75% of them have seen at least a 50% decrease in test execution time. The lion’s share of this figure is thanks to AI-powered software testing. So if many proven tech professionals invest in AI's reliability, why should you hesitate? We mean, you probably should, but the kick-the-tires approach is king.
Key takeaway: The AI revolution didn’t happen overnight; Artificial Intelligence went through all maturing stages to become a robust and reliable solution for software development challenges.
How OwlityAI disproves the myths and delivers value
What do those misconceptions about AI mean? That means people have not changed for the recent century. Industrial revolution? Won’t work. New business approaches and financial models? Not sure. Science breakthroughs? Ain’t come off.
And now let’s look up the facts on OwlityAI’s example.
It enhances human creativity and decision-making:
OwlityAI automates 97+% of your testing tasks and allows the team to focus on exploratory and usability testing. Seems like a mature understanding of own limitations: creativity and intuition — would hand them over to humans.
Has effortless setup:
A copy-and-paste approach. You just paste the link to your web app, and OwlityAI starts working. Beginner-friendly design, intuitive interfaces, and comprehensive guides ensure seamless integration into existing workflows.
Aces complexity:
OwlityAI autonomously scans, prioritizes testing areas, and manages even the most intricate applications. It flexibly adapts to dynamic environments and finds edge cases with great precision.
Reduces costs by at least 93%:
OwlityAI offers flexible pricing. The vendor’s objective is to make advanced AI testing accessible and to multiply robust smooth software. That’s why the product changes the parity: it delivers a strong return on investment with minimal manual efforts.
Measures outcomes, provides clear-cut reports:
The tool maintains and updates the current test suites library, identifying flaky tests and ensuring accurate results of working suites. After every cycle, you’ll receive a detailed and actionable report. Do you feel that sometimes you are lost in your testing moves and decisions? No longer.
Bottom line
We are witnessing how AI in software testing is changing our usual and sticky approaches step by step. Many testers proved that the testing cycle can be fast and efficient. They proved that you can spend an affordable amount on improving your testing processes while receiving the best quality.
Although some AI-powered testing myths still exist, we hope this debunking material will help you navigate the right development path weeding out all the buzz.
If you are ready to start your best software testing experience ever, hit the button below or contact us directly.
Monthly testing & QA content in your inbox
Get the latest product updates, news, and customer stories delivered directly to your inbox